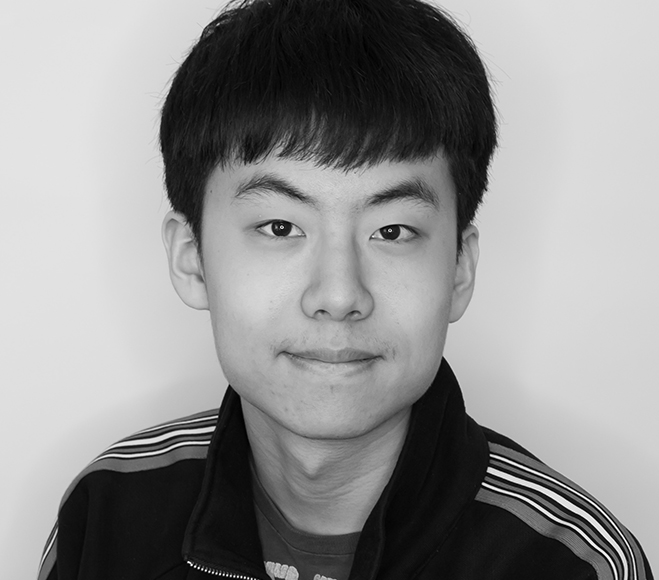
Hi there!
I'm a third year PhD student at UC San Diego in the Center for Visual Computing, advised by Prof. Tzu-Mao Li and Prof. Ravi Ramamoorthi. My research interests are in improving light transport algorithms for forward and inverse rendering, and exploring their applications. At the moment, I'm particularly interested in exploring various problems in differentiable and inverse rendering, as well as efficient sampling and denoising methods for real-time rendering. I'm also interested in GPUs and have written plenty of other software.
I received my BASc in Computer Engineering at UBC, where I worked remotely with Prof. Toshiya Hachisuka and Prof. Derek Nowrouzezahrai on efficient sampling for real-time rendering. I also worked with Prof. Tor Aamodt on improving ray tracing acceleration on GPUs.
In my free time, I enjoy cooking dishes from all around the world.
Feel free to send me an email if you'd like to chat!
Recent Publications
See more →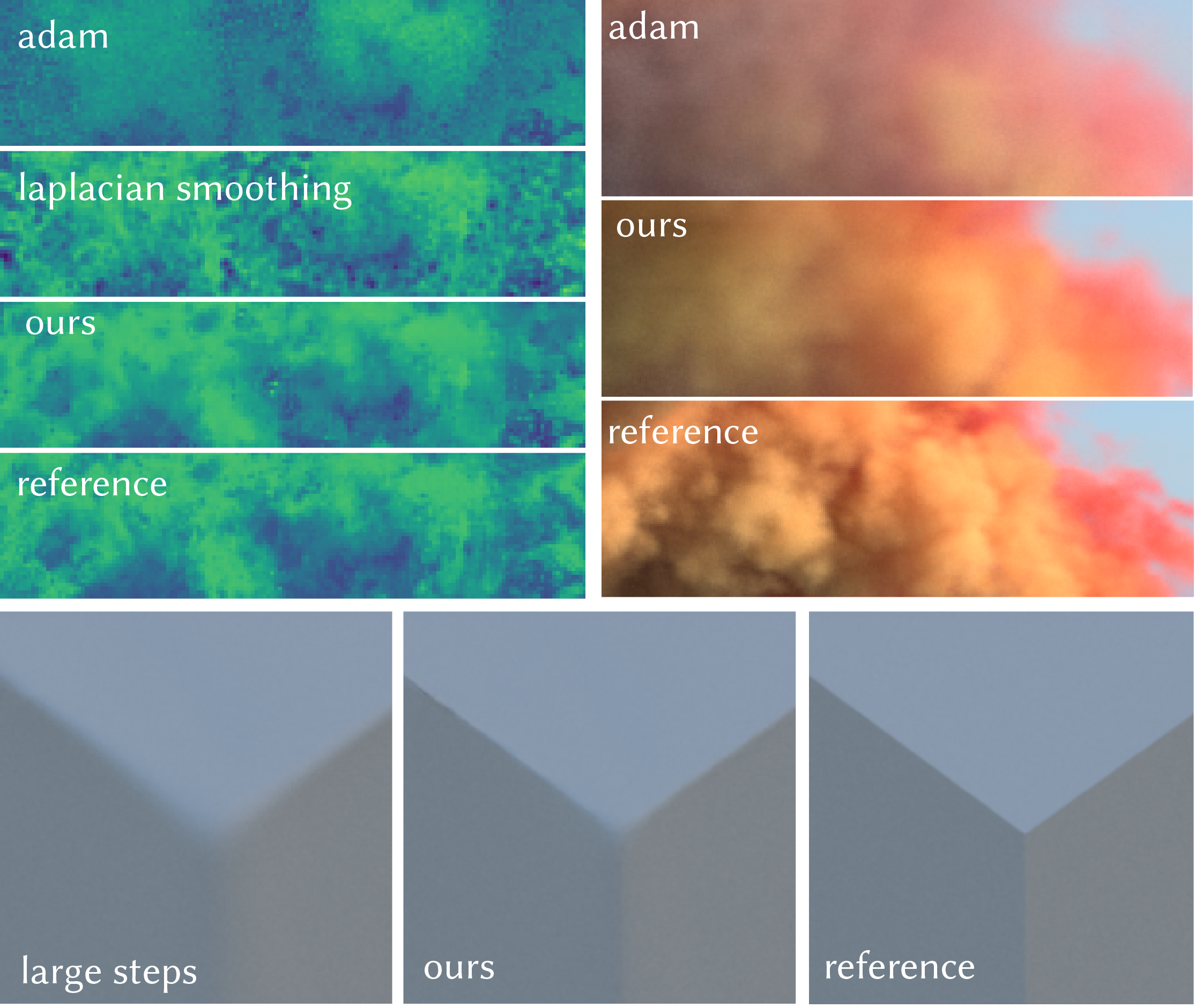
Spatiotemporal Bilateral Gradient Filtering for Inverse Rendering
Wesley Chang*, Xuanda Yang*, Yash Belhe*, Ravi Ramamoorthi, and Tzu-Mao Li
*Denotes equal contribution
SIGGRAPH Asia 2024 (Conference Track)
We introduce a spatiotemporal optimizer for inverse rendering which combines the temporal filtering of Adam with spatial cross-bilateral filtering to enable higher quality reconstructions in texture, volume, and geometry recovery.
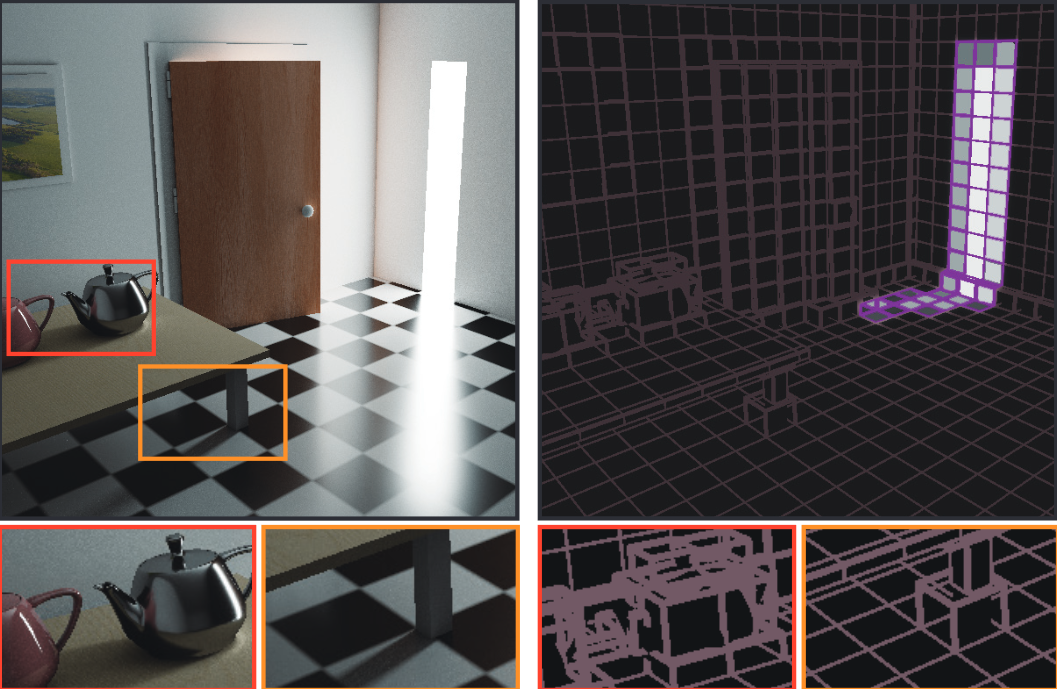
Real-Time Path Guiding Using Bounding Voxel Sampling
Haolin Lu, Wesley Chang, Trevor Hedstrom, and Tzu-Mao Li
ACM Transactions on Graphics (Proceedings of SIGGRAPH 2024)
We propose a real-time path guiding method, Voxel Path Guiding (VXPG), that significantly improves fitting efficiency under limited sampling budget. We show that our method can outperform other real-time path guiding and virtual point light methods, particularly in handling complex dynamic scenes.
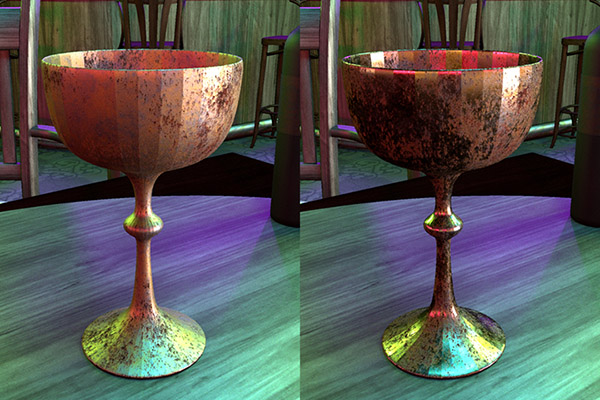
Parameter-space ReSTIR for Differentiable and Inverse Rendering
Wesley Chang, Venkataram Sivaram, Derek Nowrouzezahrai, Toshiya Hachisuka, Ravi Ramamoorthi, and Tzu-Mao Li
SIGGRAPH North America 2023 (Conference Track)
Physically-based inverse rendering algorithms that utilize differentiable rendering typically use gradient descent to optimize for scene parameters such as materials and lighting. We observe that the scene often changes slowly from frame to frame during optimization, similar to animation, and therefore adapt temporal reuse from ReSTIR to reuse samples across gradient iterations and accelerate inverse rendering.